MedalioNER
Local and ultra-lightweight AI model for extracting clinical information from medical documentation.
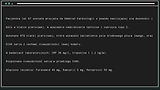
MedalionNER is a Named Entity Recognition (NER) system designed specifically for processing Polish-language medical documentation. Trained on 50,000 anonymized clinical notes, it accurately extracts key clinical information from unstructured texts — with performance on par with human experts.
What MedalioNER extracts
Diseases and Diagnoses
(e.g. myocardial infarction, type 2 diabetes
Test Results
(e.g. CRP: 42 mg/L, chest X-ray: left lower lobe opacity)
Medications
(both brand names and active substances, e.g. Paracetamol, Enalapril)
Anatomical Terms
(e.g. left kidney, thyroid gland)
Symptoms
(e.g. chest pain, fever, shortness of breath)
Departments & Specialties
(e.g. Emergency, Neurology, Cardiology Unit)
Medical Procedures
(e.g. abdominal ultrasound, gastroscopy, knee replacement)
Demographics & Identifiable Information
(e.g age, weights, name, surname)
Use Cases
Integration with HIS and EHR systems
-
Automatic extraction and population of structured fields from free-text notes
-
Filtering and retrieval of patient cases by diagnosis, procedure, or medication
-
Accelerated billing support via automatic code suggestions (ICD-10, ICD-9, ATC)
Scientific research & cohort studies
-
High-volume clinical data extraction for retrospective analysis
-
Efficient patient selection based on complex criteria (e.g. age > 65 + dyspnea + ACE inhibitors)
-
Anonymization of records for GDPR-compliant data sharing
-
Trend analysis of treatments, outcomes, and adherence to clinical guidelines
Regulatory compliance & data protection
-
Automatic detection and masking of sensitive information (names, IDs, dates)
-
Fully local processing — no data leaves your infrastructure
-
Audit support and quality assurance automation